Assisting in the Search for Dark Matter Evidence
A Deep Learning Approach to Particle Identification for the AMS Electromagnetic Calorimeter
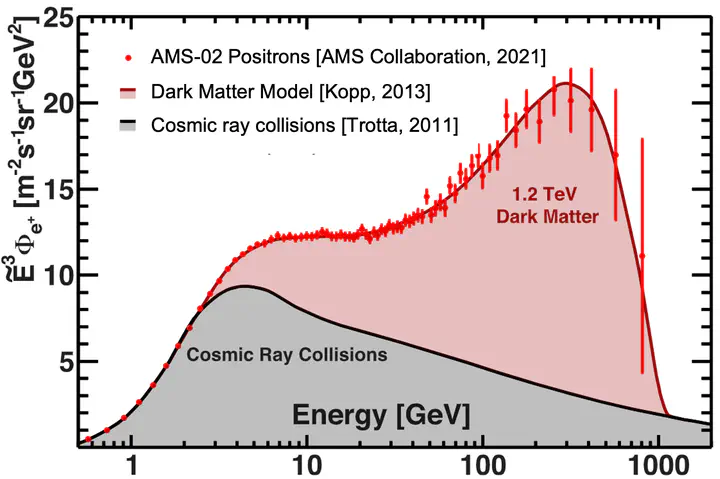
The Alpha Magnetic Spectrometer (AMS) is a high-precision particle detector onboard the International Space Station containing six different subdetectors. Its main objectives include searching for antimatter (such as positrons), investigating dark matter, and analyzing cosmic rays. Of the six subdetectors, the Electromagnetic Calorimeter (ECAL), is used to measure the energy of cosmic-ray electrons and positrons and to differentiate these particles from cosmic-ray protons up to TeV energy.
In the past, AMS measured the flux of cosmic positrons up to around 1 TeV (AMS Collaboration, 2021). They discovered that the amount cannot be explained simply by cosmic ray collisions (Trotta, 2011), and that the excess production of positrons were better explained by a dark matter model (Kopp, 2013) (see above figure). This was an important contribution towards finding evidence for dark matter. However, the story hasn’t stopped there.
Our goal with this project is to push beyond the 1 TeV energy range to learn more about highly energetic cosmic rays and attain more evidence for dark matter. We intend to do this by employing modern vision-based deep learning models, such as Residual Neural Networks and Convolutional Vision Transformers, to improve the ECAL’s background proton rejection and identify rare, high-energy cosmic positrons.
For more information, please see my published master’s thesis.